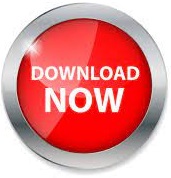

Third, algorithmic trading provides liquidity for market participants contributing to friction free transactions. Arbitrage trading contributes on the equilibrium between the spot market and futures market as well as on the price discovery.
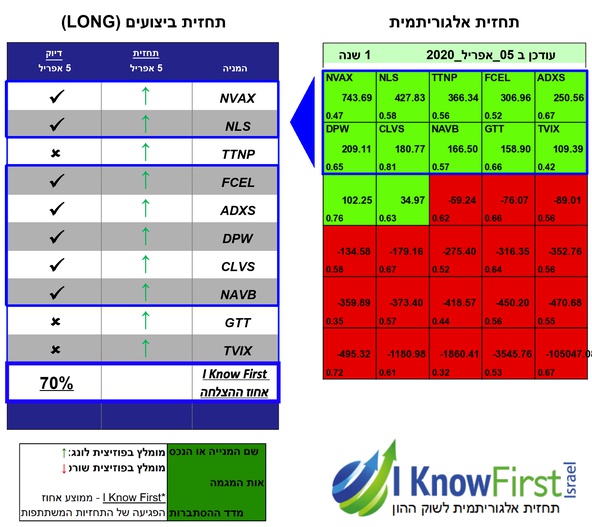
Second, an algorithmic trading also increases the operation efficiency of a stock market. First, the study results indicate that algorithmic trading contributes to the reduction in asymmetric volatility, which causes inefficiency of information in a stock market. Based on an empirical analysis of the Korean stock market, this study discovered the positive impact of algorithmic trading on stock market efficiency at three-fold. Algorithmic trading enables investors to trade stocks through a computer program without the need for human interventions. This research specifically focused on algorithmic trading. The main purpose of this study is laid on examining the role of IT based stock trading on financial market efficiency. The rapid development of information technology has changed the dynamics of financial markets. This paper proposes referential data base for the stock market prices without compromising efficiency and accuracy for market forecasting methods. Referential data base is one of the answers to this problem. Large amount of space, time, power etc have been utilized to store and process these repetitive data. Fractal behavior of the market shows repetition of the stock prices again and again. Because of acute scarcity of natural resources, processing of the stock market data requires green methodologies which contribute to save energy, power, time, space etc. Irrespective of the nature of the event and its format, market requires data integration of all kind. Data required for the stock market decision making process is generated from every event and all events have its own impact on the market. Investments solely depend upon efficiency and accuracy of the data. Changing data format and its behavior requires a new methodology to handle and integrate. Forecasting of the market is not only based on the prices of the stocks but also on other integrated information like socio-economic factors, prices, politics etc. Traditional database methodology has been used in the Indian stock market. It also tries to calculate its green effect. It also unearth the questions arises after integer value and referential value databases. This paper discusses the database model for stock market as a case study of Indian stock market and proposes a green database model. We already discussed the database based on integer value and referential value but green database model is till need some improvements. This paper is the continuation of our previous work of green database model for stock market. In market is relatively less mature than develop market and due it, large unplanned and unused data had been stored in the database of intermediaries and other stack holders. Repetition of data requires resources and it leads to the wastage of cost, energy, power, space etc. Due to this phenomenon, same data is being deposited at different places and repeated again and again. In the era of global village, stack holders and participators in stock market is developing its own repositories. Mammoth data is being created in stock market round the globe.
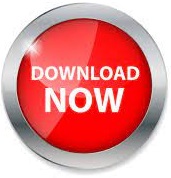